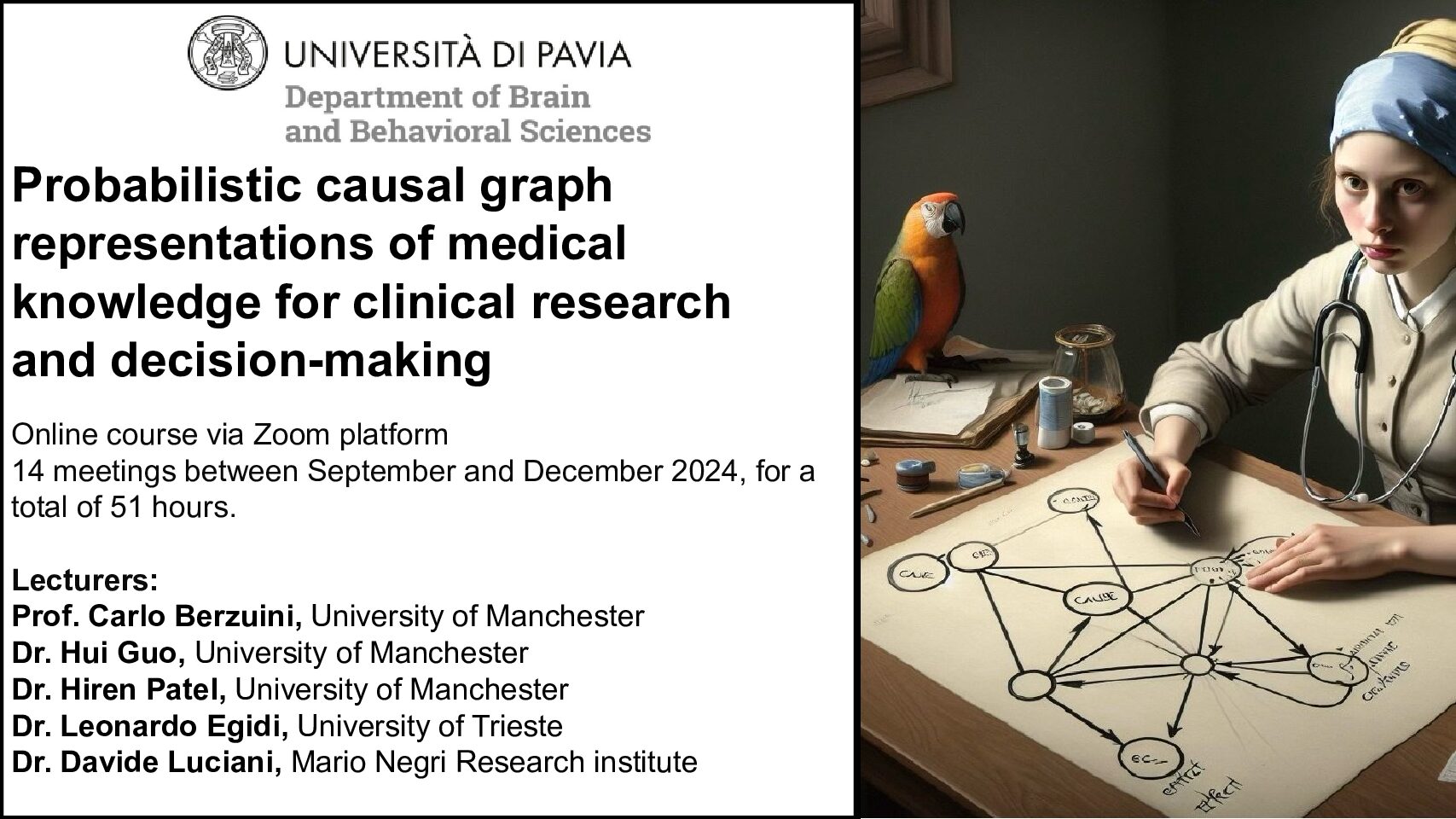
From September to December 2024 the course “Probabilistic causal graph representations of medical knowledge for clinical research and decision-making” will be held online on the Zoom platform.
To register to the course, please fill the form at the following link:
https://docs.google.com/forms/d/1Xk1gp_M79SOPPLVziFRUMau_Ys0sfZB30MwDReucL80/edit
Lecturers will be Prof. Carlo Berzuini, Dr. Hui Guo, Dr. Hiren Patel, Dr. Leonardo Egidi and Dr. Davide Luciani. The course will be held online using Zoom platform. It is divided into theoretical lessons and practical exercises on the computer using R. Registrations must be made by 31 August 2024.
14 meetings: 16-17-18 September 2024, 14-15-16 October 2024, 4-5-6, 25-26-27 November 2024, 11-12 December. 5 modules, for a total of 51 hours.
Registration Fees:
Students € 500
Academics € 800
Non-Academics € 1000
For the first 4 modules only, it is possible to register for individual modules at a cost of 250 € /module.
Scientific Committee and Organizers:
Carlo Berzuini, Luisa Bernardinelli, Teresa Fazia, Andrea Nova.
PROGRAM:
MODULE 1:
DATE: 16-17-18 September 2024
THE CHALLENGES OF CAUSAL ANALYSIS: A STATISTICAL VIEWPOINT
INSTRUCTOR: Carlo Berzuini
TOPICS:
- Prediction vs causation
- Experimental vs observational
- Examples
CAUSALITY IN MEDICINE
INSTRUCTOR: Davide Luciani
TOPICS:
- From the origins to Evidence Based Medicine
- Personalized Medicine
KNOWLEDGE REPRESENTATION AND INFERENCE VIA CAUSAL GRAPHS: THE THEORY
INSTRUCTORS: Carlo Berzuini and Hui Guo
TOPICS:
- Simple examples of causal graph in medicine
- Integrating knowledge from independent studies via the graph
- Directed acyclic graphs (DAGs)
- Probabilistic interpretation of the DAG
- Introducing causality in the DAG
- Introducing decision in the DAG
- Formulating the questions
- Identification
- Getting the answers : propensity scoring instrumental variable analysis
- Application examples
MODULE 2:
DATES: 14-15-16 October 2024.
INTRODUCTION TO BAYESIAN INFERENCE
INSTRUCTOR: Leonardo Egidi
TOPICS:
- Models, Probability
- Bayes Rule
- Conjugate Models
- MCMC Methods
- Practical Examples
MODULE 3:
DATES: 4-5-6 Novembre 2024.
PROBABILISTIC LANGUAGES FOR MODELLING AND COMPUTATION IN BAYESIAN INFERENCE
INSTRUCTOR: Leonardo Egidi
TOPICS:
- Stan: use and interfaces.
- Generative models in Stan: the prior predictive distribution.
- Inference and convergence measures: the Gelman-Rubin statistics, the effective sample size.
- Hamiltonian Monte Carlo: quick sketch of the algorithm.
- Model checking: posterior predictive checks.
- Model comparisons: leave-one-out cross-validation, model stacking.
- Related packages: rstanarm, cmdstan, bayesplot, loo, shinystan.
- Applications: clinical trials data, social science data, single-cell data for genomics.
MODULE 4:
DATES: 25-26-27 November 2024.
FOCUS ON DIAGNOSIS, WITH AN APPLICATION IN EMERGENCY MEDICINE
INSTRUCTOR: Davide Luciani
TOPICS:
- DAG structures and diagnostic reasoning
- blurred boundaries and granularity of diagnostic domains
- from simple to complex types of diagnostic inferences
- Support of decision in diagnostic process
- diagnostic judgements and satisfaction bias
- the value of information
- optimal diagnostic strategies
- Critical issues in elicitation of medical knowledge.
- causal architecture of biomedical relations
- conditional models choice
- quantitative parameters
- What can be learnt from clinical data
- coping with missing values
- addressing data selection
- Structural criticism
- structural inadequacies detection tools
- what evidence supports structural refinement
- the continuous improvement of clinical practice
MODULE 5:
DATES: 11-12 December 2024
APPLICATIONS IN THE STUDY OF UNSOLVED THERAPEUTIC DECISION PROBLEMS AND CLINICAL VARIABILITY
INSTRUCTOR: Carlo Berzuini
TOPICS:
- Eliciting a causal graph for dynamic decisions in the management of hospitalised patients
- Selection bias
- Problems in the analysis and interpretation of variability in clinical practice
- Identifying areas of high decisional variability and insufficient evidential support in the therapeutic process
- Combining evidence from experimental and observational data for improved guidance in the therapy
FOCUS ON THERAPY, WITH AN APPLICATION IN NEUROSURGERY
INSTRUCTOR: Hiren Patel
TOPICS:
- The clinical problem: the important questions
- Inadequacy of clinical trials: need of a causal graph approach
- Knowledge elicitation and formulation of a DAG model for dynamic treatment decision in subarachnoid harmorrhage (SAH)
- Exploring the causes of clinical outcome variation across institutions
- Estimating the effects of interventions
- Myopic vs non-myopic optimization
- Missing data management: imputation model larger than inference model
Professor Carlo Berzuini (Scientific co-director) served as a Professor of Biostatistics at the University of Pavia, Italy, until 2008. At that time, he relocated to Cambridge, where he held the position of Project Leader at the Cambridge Biostatistics Unit of the Medical Research Council and the Faculty of Mathematics at Cambridge University. In 2011, he joined the University of Manchester as a Research Professor of Biostatistics, achieving Emeritus status in 2023. His groundbreaking contributions encompass various fields, including early work in 1976 on mathematical models for diagnosing metabolic diseases. He pioneered the use of graphical modelling and Markov Chain Monte Carlo (MCMC) methods of inference in medical monitoring (1992, collaboration with D. Spiegelhalter), introduced the first practical method for joint modeling of longitudinal and time-to-event data in 1996 and, at the same time, he engaged in Bayesian prediction of epidemic trends over multiple time scales. In partnership with David Clayton, he proposed a Bayesian solution of the unidentifiability problem in age-period-cohort modeling. His collaboration with Sarah Teichmann in 2004 resulted in predicting protein-protein affinity through the innovative concept of “supradomain.” Alongside Wally Gilks in 1997, he invented the class of Sequential Markov Chain Monte Carlo methods of inference (1500+ citations), now widely applied in data science, epidemic prediction, real-time target identification, medical monitoring, and beyond. More recently, he received a Scientific Award from the Cambridge Isaac Newton Institute for his research in causal inference and secured a Research Grant from the UK Medical Research Council for collaborative work with Philip Dawid on causative interaction and the assessment of dynamic treatment regimes. He was lead editor (jointly with Philip Dawid and Luisa Bernardinelli) of a landmark book in Causal Inference (2012) and the lead organizer of the first international Conference in Causal Inference held in Europe (2009, Faculty of Mathematical and Physical Sciences of the University of Cambridge). Since 2011 he has led a research group (later joined by Dr. Hui Guo) working in particular on Bayesian extensions of Mendelian Randomization methods, modelling of pain perception (collaboration with Deborah Talmi and Hui Guo) and currently collaborating with Hiren Patel on a multicentre observational study inq the treatment of subarachnoid haemorrhage. Besides his methodological contributions, he has an extensive interdisciplinary research record that underscores his multifaceted contributions to various scientific domains.
Dr Hui Guo is a Reader in Biostatistics and the Head of the Centre for Biostatistics at the University of Manchester. She studied for a PhD on Statistical Causal Inference and Propensity Analysis (supervisor: Professor Philip Dawid) and then worked as a Research Associate at the University of Cambridge. Her postdoctoral research (supervisor: Dr Chris Wallace) focused on the development and application of statistical methodologies with the aim of understanding the genetics and mechanisms of common complex diseases. Hui’s research expertise is in statistical causal inference from observational studies. Her current work involves the identification of causal genes for clinical outcomes from large-scale studies. She is also interested in predictive models, genetic causal pathways and disease networks using multi-omics data.
Dr Leonardo Egidi is senior assistant professor (RTD-B) at the ‘Bruno de Finetti’ Department of Business, Economics, Mathematics and Statistics (DEAMS) of the University of Trieste. He teaches Courses in Advanced Statistics, Statistics and Machine Learning, and Bayesian Statistics in the same university. He obtained his PhD in Statistics from the Department of Statistical Sciences of the University of Padova, and during his PhD he was a visiting fellow at the Department of Statistical Sciences of Columbia University, New York. He is a proficient R package developer and his research topics include: Bayesian robust prior specification, hierarchical modeling, clinical trials analysis, clustering, replication crisis in science, probabilistic programming language, single cell data, and sports modeling. In 2023, he became the Principal Investigator (PI) of a PRIN project focused on sports modeling. He provides statistical consultancy to small firms and hospitals and he has several research collaborations, among the others with University of Pavia, Columbia University, Athens University of Economics and Business. Actually, he is the vice-chair of the ISI Special Interest Group in Sports Statistics. He is an affiliated member of the Italian Statistical Society (SIS), the International Society for Bayesian Analysis (ISBA) and the International Statistical Institute (ISI). He acts as referee for many high standard statistical journals, such as Journal of the Royal Statistical Soiety (A and C) , Biostatistics, and Statistics and Probability Letters.
Dr Hiren Patel (PhD FRCS). Clinically and through his research, Manchester-based Mr Patel has developed an expertise in managing patients with all aspect of brain injury, and he has published widely on the subject. His observations published in the Lancet have changed the way head injuries are managed in the UK, and he led the change of how this care is delivered for patients in Greater Manchester. Besides his significant research grant income, he has set up and runs the National SAH outcome database and is a member of a national team looking at improving outcome after ICH.
Dr Davide Luciani (Dipartimento di Ricerca Ambiente e Salute, Mario Negri Research Institute, Milan, Italy; davide.luciani@marionegri.itsi ) has a degree in Medicine and Surgery from the University of Bologna. He has used his experience from years of medical practice for investigating and formalizing clinical judgment and decision making. To this end, he has collaborated with academic experts in Statistics and Computer Science, in Italy, at the University College of London and at the Department of Computer Science of Aalborg. Since 2005 he has led the Clinical Knowledge Engineering Unit at the Mario Negri Institute, where he has worked with real medical data to develop probabilistic expert systems based on graphical models, Markov chain Monte Carlo methods of estimation and knowledge acquisition methods. Among his achievements is the probabilistic expert system BayPAD, that currently covers hundreds of diagnoses of interest in the Emergency Departments, where patients are typically admitted with generic symptoms.